Evaluation¶
Slideflow includes several tools for evaluating trained models. In the next sections, we’ll review how to evaluate a model on a held-out test set, generate predictions without ground-truth labels, and visualize predictions with heatmaps.
Evaluating a test set¶
The slideflow.Project.evaluate()
provides an easy interface for evaluating model performance on a held-out test set. Locate the saved model to evaluate (which will be in the project models/
folder). As with training, the dataset to evaluate can be specified using either the filters
or dataset
arguments. If neither is provided, all slides in the project will be evaluated.
# Method 1: specifying filters
P.evaluate(
model="/path/to/trained_model_epoch1",
outcomes="tumor_type",
filters={"dataset": ["test"]}
)
# Method 2: specify a dataset
dataset = P.dataset(tile_px=299, tile_um='10x')
test_dataset = dataset.filter({"dataset": ["test"]})
P.evaluate(
model="/path/to/trained_model_epoch1",
outcomes="tumor_type",
dataset=test_dataset
)
Results are returned from the Project.evaluate()
function as a dictionary and saved in the project evaluation directory. Tile-, slide-, and patient- level predictions are also saved in the corresponding project evaluation folder, eval/
.
Generating predictions¶
For a dataset¶
slideflow.Project.predict()
provides an interface for generating model predictions on an entire dataset. As above, locate the saved model from which to generate predictions, and specify the dataset with either filters
or dataset
arguments.
dfs = P.predict(
model="/path/to/trained_model_epoch1",
filters={"dataset": ["test"]}
)
print(dfs['patient'])
patient ... cohort-y_pred1
0 TCGA-05-4244-01Z-00-DX1... ... 0.032608
1 TCGA-05-4245-01Z-00-DX1... ... 0.216634
2 TCGA-05-4249-01Z-00-DX1... ... 0.000858
3 TCGA-05-4250-01Z-00-DX1... ... 0.015915
4 TCGA-05-4382-01Z-00-DX1... ... 0.020700
.. ... ... ...
936 TCGA-O2-A52S-01Z-00-DX1... ... 0.983500
937 TCGA-O2-A52V-01Z-00-DX1... ... 0.773328
938 TCGA-O2-A52W-01Z-00-DX1... ... 0.858558
939 TCGA-S2-AA1A-01Z-00-DX1... ... 0.000212
940 TCGA-XC-AA0X-01Z-00-DX1... ... 0.632612
Results are returned as a dictionary of pandas DataFrames (with the keys 'tile'
, 'slide'
, and 'patient'
for each level of prediction) and saved in the project evaluation directory, eval/
.
For a single slide¶
You can also generate predictions for a single slide with either slideflow.slide.predict()
or slideflow.WSI.predict()
.
import slideflow as sf
slide = '/path/to/slide.svs'
model = '/path/to/model_epoch1'
sf.slide.predict(slide, model)
array([0.84378019, 0.15622007])
The returned array has the shape (num_classes,)
, indicating the whole-slide prediction for each outcome category. If the model was trained with uncertainty quantification, this function will return two arrays; the first with predictions, the second with estimated uncertainty.
Heatmaps¶
For a dataset¶
Predictive heatmaps can be created for an entire dataset using slideflow.Project.generate_heatmaps()
. Heatmaps will be saved and exported in the project directory. See the linked API documentation for arguments and customization.
P.generate_heatmaps(model="/path/to/trained_model_epoch1")
For a single slide¶
slideflow.Heatmap
provides more granular control for calculating and displaying a heatmap for a given slide. The required arguments are:
slide
: Either a path to a slide, or aslideflow.WSI
object.model
: Path to a saved Slideflow model.
Additional keyword arguments can be used to customize and optimize the heatmap. In this example, we’ll increase the batch size to 64 and allow multiprocessing by setting num_processes
equal to our CPU core count, 16.
heatmap = sf.Heatmap(
slide='/path/to/slide.svs',
model='/path/to/model'
batch_size=64,
num_processes=16
)
If slide
is a slideflow.WSI
, the heatmap will be calculated only within non-masked areas and ROIs, if applicable.
from slideflow.slide import qc
# Prepare the slide
wsi = sf.WSI('slide.svs', tile_px=299, tile_um=302, rois='/path')
wsi.qc([qc.Otsu(), qc.Gaussian()])
# Generate a heatmap
heatmap = sf.Heatmap(
slide=wsi,
model='/path/to/model'
batch_size=64,
num_processes=16
)
If slide
is a path to a slide, Regions of Interest can be provided through the optional roi_dir
or rois
arguments.
Once generated, heatmaps can be rendered and displayed (ie. in a Jupyter notebook) with slideflow.Heatmap.plot()
.
heatmap.plot(class_idx=0, cmap='inferno')
Insets showing zoomed-in portions of the heatmap can be added with slideflow.Heatmap.add_inset()
:
heatmap.add_inset(zoom=20, x=(10000, 10500), y=(2500, 3000), loc=1, axes=False)
heatmap.add_inset(zoom=20, x=(12000, 12500), y=(7500, 8000), loc=3, axes=False)
heatmap.plot(class_idx=0, mpp=1)
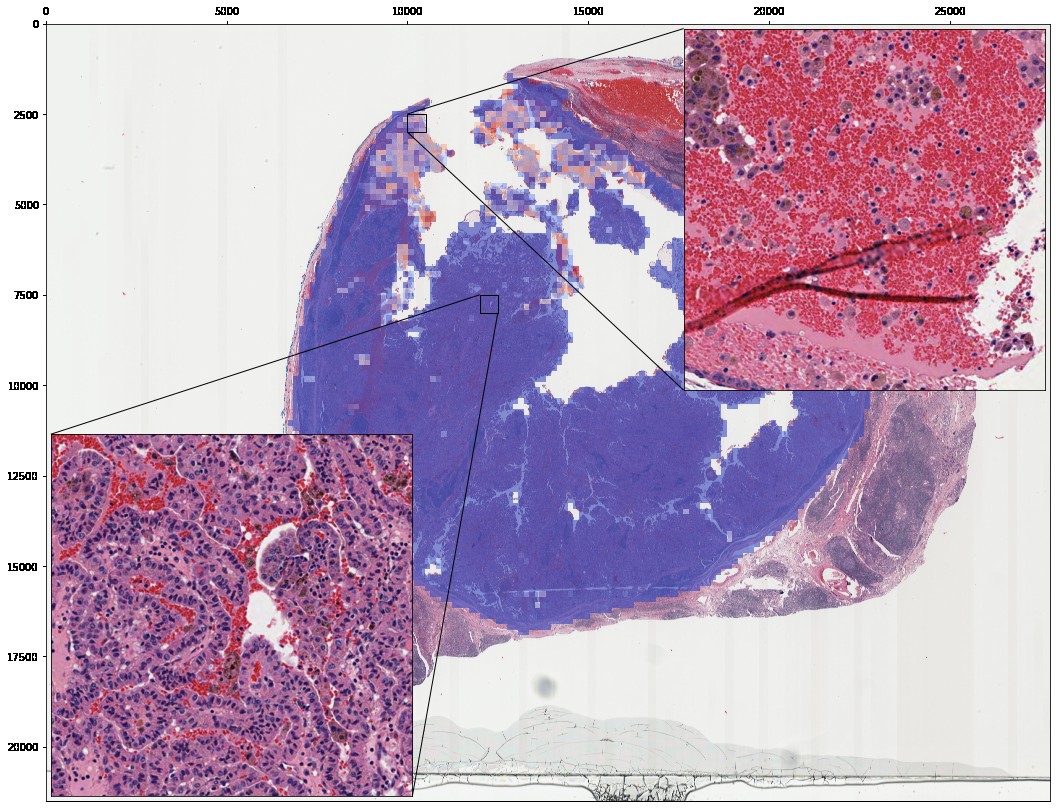
Save rendered heatmaps for each outcome category with slideflow.Heatmap.save()
. The spatial map of predictions, as calculated across the input slide, can be accessed through Heatmap.predictions
. You can save the numpy array with calculated predictions (and uncertainty, if applicable) as an *.npz file using slideflow.Heatmap.save_npz()
.